This blog demonstrates how to migrate data from different database to databricks.
Migrating snowflake database table into databricks:

The steps below describe how to migrate data from snowflake table to a databricks table.
Step 1:
Create a new snowflake account or use an existing account.
Step 2:
Create a table in snowflake.
CREATE OR REPLACE TABLE MAIN.CITY(CITY_ID BIGINT,CITYNAME STRING,PINCODE INT,COUNTRY STRING);
Below query is used to insert values in the table,
INSERT INTO MAIN.CITY VALUES
(10020,'NEW DELHI',110001,'INDIA'),
(10021,'MUMBAI',400001,'INDIA'),
(10022,'CHENNAI',600028,'INDIA'),
(10023,'LONDON',400706,'UNITED KINGDOM'),
(10024,'PARIS',403101,'FRANCE'),
(10025,'DUBAI',600017,'UNITED ARAB EMIRATES'),
(10026,'SINGAPORE',395004,'SOUTHEAST ASIA'),
(10027,'NEW YORK',360005,'UNITED STATES OF AMERICA'),
(10028,'TOKYO',533101,'JAPAN');
To view the table, use below select query in snowflake,
SELECT * FROM MAIN.CITY;
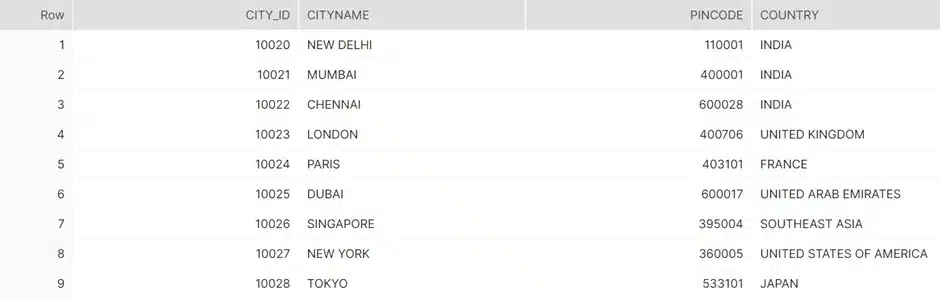
Step 3:
Provide the username, URL (hostname), password, warehouse name and database name to connect snowflake to databricks.
url_1="hostname"
user="snowflake_username"
password="snowflake_password"
database="database_name"
sfWarehouse="warehouse_name"
sfOptions = {
"sfUrl": url_1,
"sfUser": user,
"sfPassword": password,
"sfDatabase": database,
"sfWarehouse": sfWarehouse
}
Note:
sfUrl, sfUser, sfPassword, and sfDatabase must be provided. And sfWarehouse can be optional.
Step 4:
As shown below, select query should be saved in a string variable. Get the data from query and store it in a dataframe using spark methods.
query=”SELECT * FROM MAIN.CITY”
df=spark.read.format("net.snowflake.spark.snowflake").options(**sfOptions).option("query", query).load()
show() is used to display the contents of the DataFrame.
df.show()
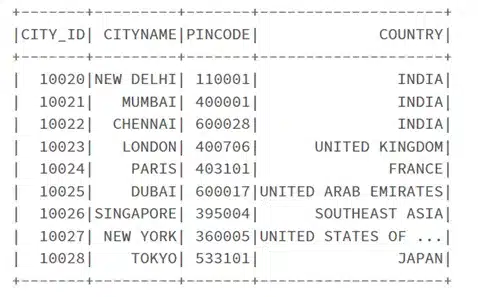
Below saveAsTable() used to save the contents of the DataFrame to a databricks table,
df.write.saveAsTable(“main.city”)
If schema is not provided, the table is stored in default schema. If schema is provided, the table will be stored in mentioned schema.
To view the schema of the table, the below command can be used in databricks sql.
DESC TABLE MAIN.CITY;
The schema of the table is shown below,
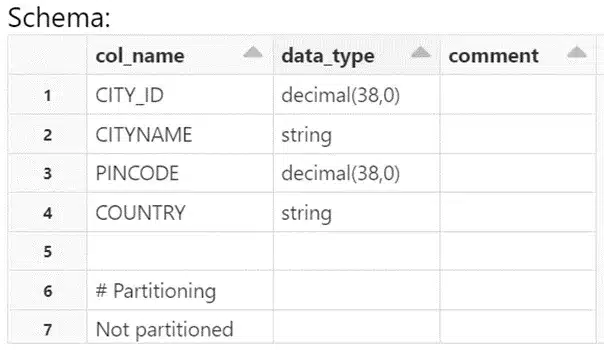
To select the table, use below command in databricks sql.
SELECT * FROM MAIN.CITY;
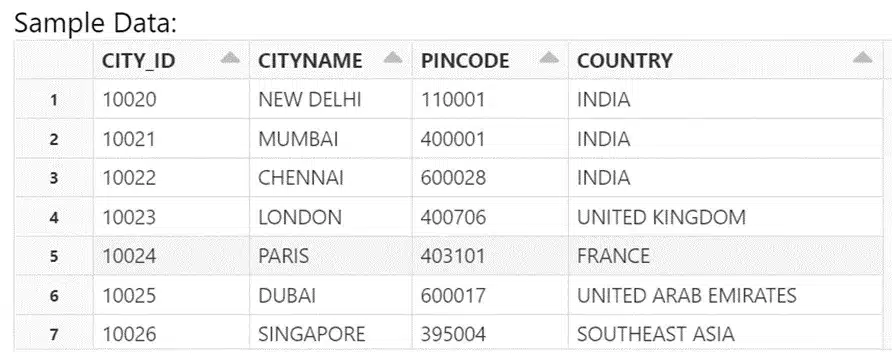
Migrating snowflake database table into databricks:

The steps below describe how to migrate data from oracle table to a databricks table.
Step 1:
Create a table in oracle database.
CREATE TABLE MAIN.LAPTOPS(LAPTOP_ID INT,LAPTOP_NAME VARCHAR(40),SPECIFICATION VARCHAR(70),OS VARCHAR(30),PRICE_IN_RUPEES INT);
Below query is used to insert values in that table,
INSERT ALL
INTO MAIN.LAPTOPS VALUES(1,'Apple 16-Inch MacBook Pro M1 Max','10-core | 3.1 GHz Processor ','Mac Monterey OS',309490)
INTO MAIN.LAPTOPS VALUES(2,'Lenovo Yoga 9i','11th Gen Intel Core i7–1185G7 | 3.00 GHz processor', 'Windows 10 Home OS',164686)
INTO MAIN.LAPTOPS VALUES(3,'Dell New XPS 13 Plus','12th Gen Intel EVO Core i7–1260P | 3.40 GHz Processor', 'Windows 11 OS',215000)
INTO MAIN.LAPTOPS VALUES(4,'Apple MacBook Air M2','Apple M2 | NA Processor',' iOS OS',139390)
INTO MAIN.LAPTOPS VALUES(5,'Acer Nitro 5','12th Gen Intel Core i7–12700H | 4.70 GHz processor', 'Windows 11 Home OS',101000)
INTO MAIN.LAPTOPS VALUES(6,'Lenovo IdeaPad Gaming 3i','12th Gen Intel Core i5–12450H | 2.0 GHz processor', 'Windows 11 Home OS',79900)
INTO MAIN.LAPTOPS VALUES(7,'Acer Swift X','AMD Ryzen 7–5800U | 1.9 GHz processor', 'Windows 11 Home OS',99999)(30),PRICE_IN_RUPEES INT);
To view the records in the table, use below select query in Oracle database,
SELECT * FROM MAIN.LAPTOPS;
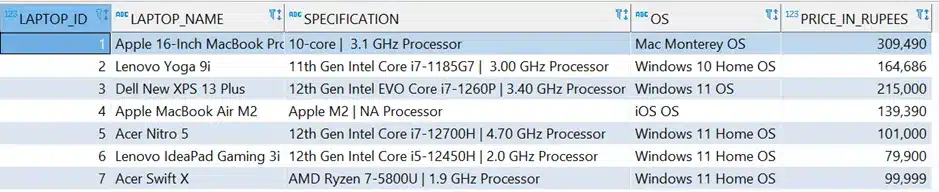
Step 2:
To install oracledb module, below pip command is required.
pip install oracledb
Step 3:
Import the module and provide Oracle database credentials as shown below,
import oracledb
user='oracle_username'
password='oracle_password'
port='port_no' #default : 1521
service_name='ORCL'
host_name='host_name of oracle database'
conn_string="{0}:{1}/{2}".format(host_name,port,service_name)
Step 4:
Establish a connection to a database using cursor () and execute a sql query using execute().
connection= oracledb.connect(user=user, password=password, dsn=conn_string)
cursor=connection.cursor()
query=cursor.execute("SELECT * FROM MAIN.LAPTOPS")
Step 5:
To extract the column names, use cursor.description as shown below,
column_names = ([i[0] for i in cursor.description])
print(column_names)
This column_names variable contains columns in the table as a list.

Step 6:
Create a dataframe with the query and column_names variable.
table_df=spark.createDataFrame(query,column_names)
table_df.show()
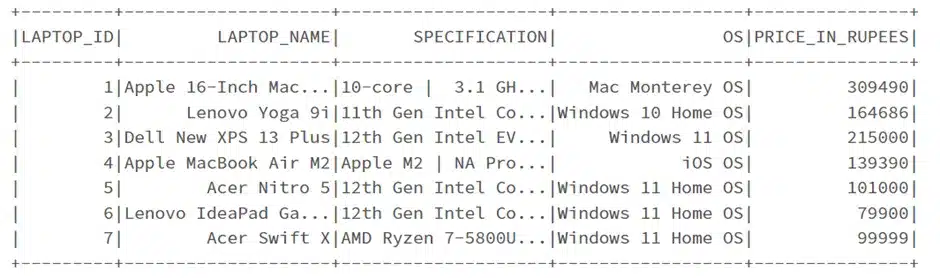
Step 7:
Create a table with saveAsTable(). If no schema is provided, the table is stored in default schema.
table_df.write.saveAsTable(“laptops”)
If schema is provided, the table will be stored in mentioned schema.
table_df.write.saveAsTable(“main.laptops”)
The schema of the table and databricks table is given below,
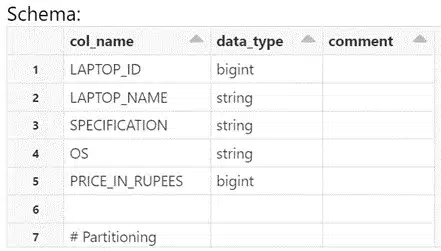
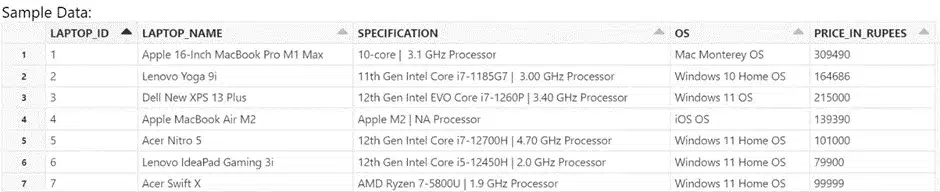
Conclusion:
At last, the tables from the Oracle and Snowflake databases are stored into another table in DataBricks by following these steps.